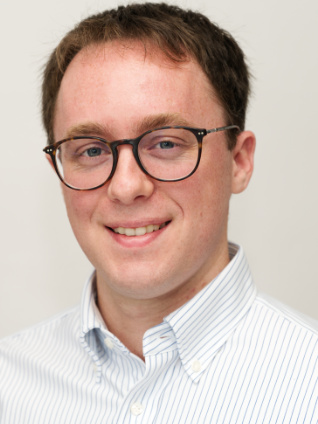
Marc-André Legault
Current projects
Nonparametric Mendelian Randomization
I'm working on the development and application of nonparametric instrumental variable estimation methods for Mendelian Randomization (MR). This is important because it allows for effect heterogeneity which is prevalent in genetics. For example, in our previous work on CETP, we have found sex and body mass index to modify substantially the genetic effect of CETP variants on a range of relevant biomarkers including atherogenic cholesterol fractions. Such effect heterogeneity is often ignored in MR, sometimes leading to bias, and the methods we are developing don't make parametric assumptions, allow for genetic effect heterogeneity and allow nonlinear relationships.
Using topic models to improve phenotyping in large cohorts
In collaboration with Dr Yue Li (McGill), we are evaluating how topic models trained on multi-modal electronic health data can improve phenotyping, especially in the context of phenome-wide association studies.
Machine-learning to improve trans-ancestry genetic prediction
Polygenic scores based on many genetic variants have demonstrated interesting predictive performance across a wide range of traits. However, it is well known that their predictive power is greatly attenuated in individuals whose genetic ancestry is not well represented in the training dataset. We are investigating whether algorithms developed for out of distribution prediction can improve polygenic prediction in admixed individuals or in individuals whose genetic ancestry was not well represented in the dataset used to develop the polygenic score.
Drug target genetics
Drugs often do their therapeutic action by modulating a protein target in the body. For example, statins, a cholesterol lowering drug, inhibit an enzyme (their drug target) responsible for the biosynthesis of cholesterol. Naturally occurring genetic variants in drug targets that have an effect on their concentration or activity may have effects that are similar to the target-modulating drugs. We can leverage this relationship to predict the safety and efficacy of new drugs under development. There are currently many statistical challenges in this field where methods to detect drug-drug interactions or to predict effect heterogeneity are of interest.
Past projects
Genetic models of CETP inhibitors
The CETP is an enzyme responsible for exchanges of cholesteryl esters between different lipoproteins. Its net effect is to increase the cholesterol carried by high density lipoproteins (with many other more subtle effects), and it plays a role in a mechanism involved in atherosclerotic plaque metabolism. This enzyme is also important because it is a drug target for a widely studied and somewhat controversial class of drugs called CETP inhibitors developed to treat coronary artery disease.
I worked on two projects aimed at better understanding the effect of a genetically predicted reduction in CETP levels:
Human genetics for drug target validation, the case of ivabradine
Ivabradine is a drug that inhibits an ion channel at the sinoatrial node in the heart called the HCN4. This channel is responsible for the spontaneous depolarization of the heart that results in its autonomous contraction. Inhibiting this channel reduces the heart rate which was expected to be beneficial in various medical conditions where the heart's energy balance is disturbed. For example, ivabradine is beneficial for the treatment of heart failure in individuals with elevated heart rate and also alleviates anginal symptoms in patients with stable angina. However, it did not protect against coronary events in patients with stable coronary artery disease without heart failure.
To see if a genetic approach could have predicted ivabradine's efficacy and safety profile, we compared a genetic model of ivabradine treatment based on genetic variants in the HCN4 gene to the results from randomized controlled trials. After accounting for comorbidities using a competing risk survival analysis model, our results recapitulated the main observations from clinical trials. We also used a Mendelian Randomization approach to further assess the effect of a heart rate reduction on heart failure an coronary artery disease outcomes.
The paper for this project is now published:
The ExPheWas browser
The ExPheWas browser allows users to browse the results of a gene-based PheWAS analysis in the UK Biobank. More than 23 million statistical association tests between protein coding genes and various phenotypes are presented. Data visualization tools and enrichment analyses are also available allowing, for example, to find drug classes that seem to share a biological basis with the phenotype. Concretely, this data visualization displays results for an enrichment analysis between disease associated genes and drug target genes from the ChEMBL database. There are many more features and interesting results which are more deeply covered in the preprint: